Performance assessment and after action review for simulation-based training
Dr Samer Hanoun, Senior Research Fellow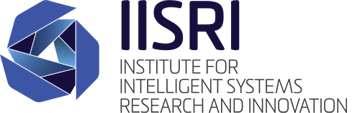
In simulation-based training, traditional methods based on subjective assessment are mostly used to evaluate performance, either through observing the trainee performance during the training exercise or by reviewing it later on a videotape.
These methods are primarily manual, time-consuming, dependent on the assessment of expert trainers and overall cognitively demanding. Additionally, post-training After Action Review (AAR) may or may not be offered, which influences the trainee self-reflection, aiming to enhance their acquired skills and learning goals.
IISRI researchers have developed automated and rigorous performance evaluation techniques to support expert trainers and instructors with AAR in simulation-based training in the medical domain. Clinical training scenarios and domain knowledge are modelled via parametrised rules associated with logical and temporal constraints to give trainers the ability to create different scenarios and tailor them to the trainees’ learning levels.
A developed framework employs temporal pattern matching of trainees’ actions against encoded domain knowledge in a rules engine to report on satisfied, violated and missed actions. These are used to identify strengths and limitations in the trainees’ applied technical skills.
Dynamic Time Warping (DTW) is augmented with optimal distance metrics to compare performance of different trainees, based on a complex set of factors such as time, action and importance. Comparison results are clustered using Support Vector Machine (SVM) into cohorts’ performance groups, to provide the ability to track the evolution in a trainee’s performance from session to session, and from one level to the next.
The developed research provides many benefits to trainers and trainees. Firstly, automated assessment of performance supports providing the trainee with immediate feedback after the training session. Secondly, the cognitive load on the trainer is reduced, allowing them to focus on observing non-technical skills such as communication, decision making and situational awareness of trainees. Thirdly, it is possible to compare trainees’ current performances with previous performances to indicate changes in skills acquired from one year to another, or from one level to the next.
The developed framework is generic, can be applied to different training domains as it provides the ability to adapt the modelled scenarios to the required complexities, learning objectives and training levels.