Safer roads through advanced driver awareness monitoring systems
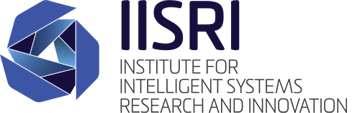
Having alert drivers is fundamental for having safe road. The purpose of this research is to monitor the driver’s behaviour using multidimensional signals and advanced machine learning technique to detect the onset of performance degradation due to the lack of concentration.
Being distracted or inattentive is the single biggest cause of road accidents in most developed countries. Drivers are often distracted due to technology-based factors such as infotainment systems, mobile phones or non-technology-based activities such as eating and drinking. Accordingly, advanced monitoring systems are required to monitor driver behaviour and generate warning alarms if lack of awareness is detected. Researchers at IISRI evaluate multidimensional datasets gathered from vehicles and drivers to determine the onset of distraction.
Physiological signals, such as perinasal electrodermal activity, heart rate, breathing rate and palm electrodermal activity are measured during simulated driving sessions. Lane deviation of the vehicle during simulation is treated as the response variable (awareness). Measured physiological signals are pre-processed to extract informative features for awareness evaluation.
These features are then passed to multiple traditional and advanced machine learning models for the purpose of binary classification and detection of the onset of distraction. The prediction results obtained indicate that machine learning algorithms are able to quickly and precisely determine whether the driver is distracted. The area under the curve for the best performing model is 0.92, which is a sign of its excellent predictive capability.
Furthermore, the research showed that physiological signals, such as palm and perinasal electrodermal activity, are the most important/informative signals for awareness evaluation. This key finding sets the minimum hardware requirements for the design of future drivers’ awareness monitoring systems which are accurate, cost effective, and computationally less demanding.